2 Audience
Identifying and managing AI risks and potential impacts β both positive and negative β requires a broad set of perspectives and actors across the AI lifecycle. Ideally, AI actors will represent a diversity of experience, expertise, and backgrounds and comprise demographically and disciplinarily diverse teams. The AI RMF is intended to be used by AI actors across the AI lifecycle and dimensions.
The OECD has developed a framework for classifying AI lifecycle activities according to five key socio-technical dimensions, each with properties relevant for AI policy and governance, including risk management [OECD (2022) OECD Framework for the Classification of AI systems | OECD Digital Economy Papers]. Figure 2 shows these dimensions, slightly modified by NIST for purposes of this framework. The NIST modification highlights the importance of test, evaluation, verification, and validation (TEVV) processes throughout an AI lifecycle and generalizes the operational context of an AI system.
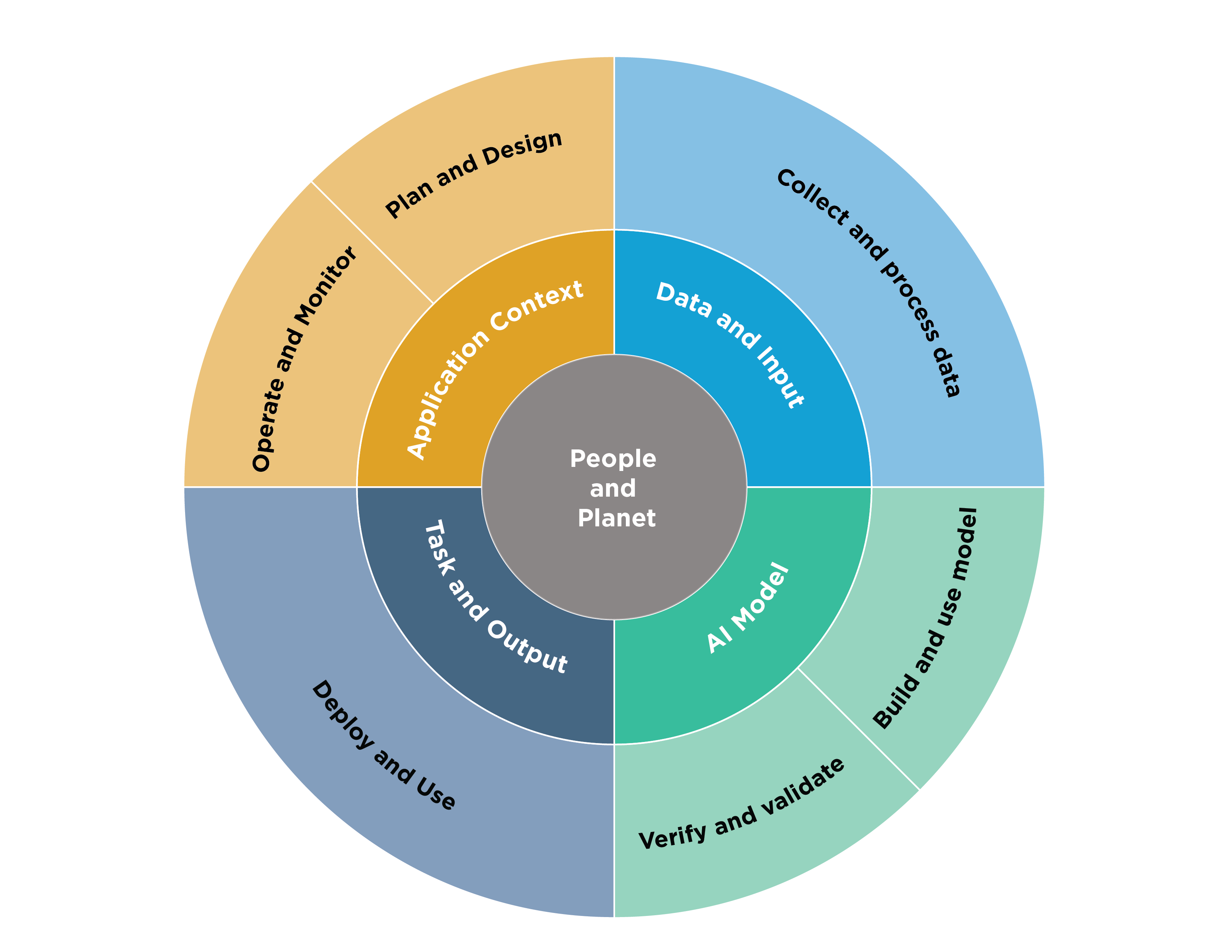
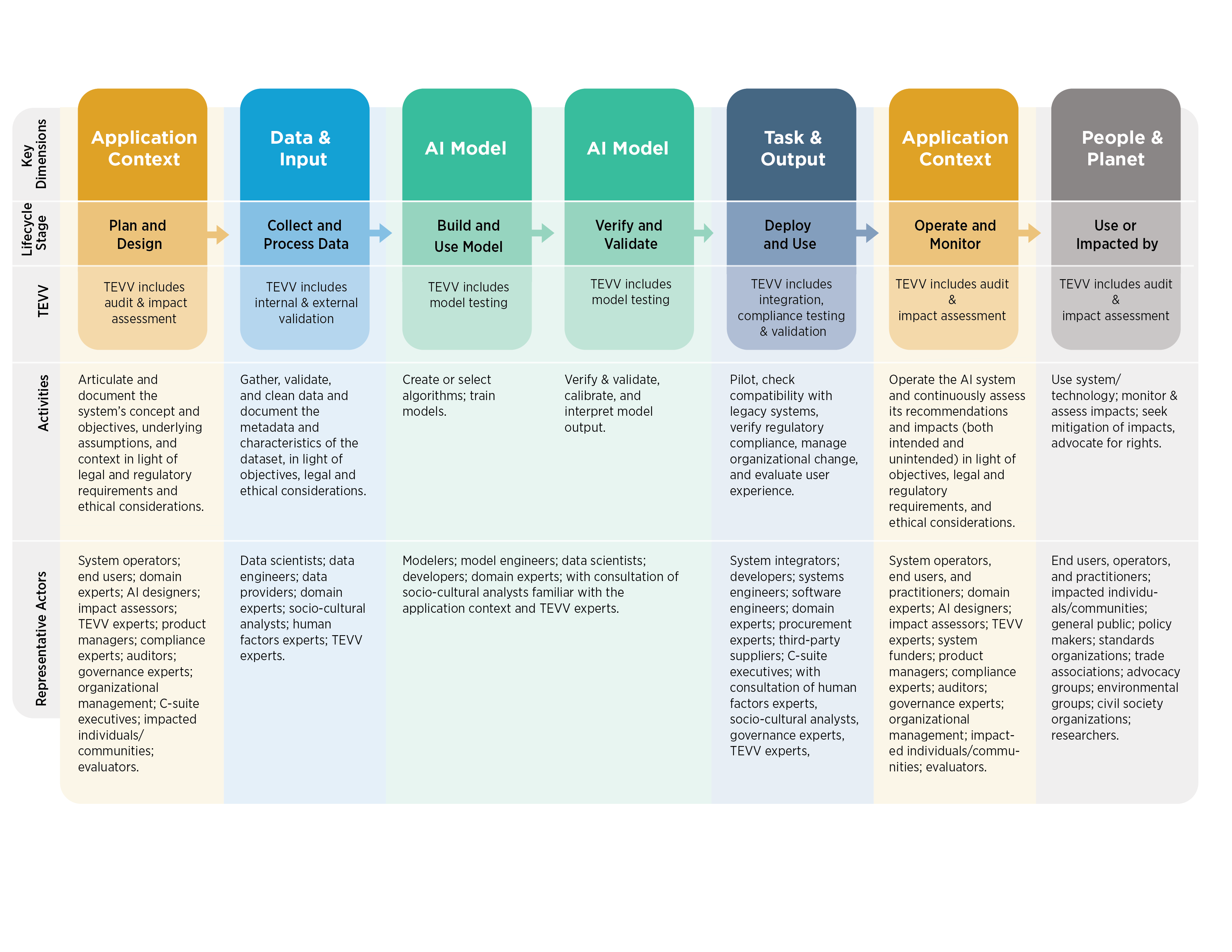
AI dimensions displayed in Figure 2 are the Application Context, Data and Input, AI Model, and Task and Output. AI actors involved in these dimensions who perform or manage the design, development, deployment, evaluation, and use of AI systems and drive AI risk management efforts are the primary AI RMF audience.
Representative AI actors across the lifecycle dimensions are listed in Figure 3 and described in detail in Appendix A. Within the AI RMF, all AI actors work together to manage risks and achieve the goals of trustworthy and responsible AI. AI actors with TEVV-specific expertise are integrated throughout the AI lifecycle and are especially likely to benefit from the Framework. Performed regularly, TEVV tasks can provide insights relative to technical, societal, legal, and ethical standards or norms, and can assist with anticipating impacts and assessing and tracking emergent risks. As a regular process within an AI lifecycle, TEVV allows for both mid-course remediation and post-hoc risk management.
The People & Planet dimension at the center of Figure 2 represents human rights and the broader well-being of society and the planet. The AI actors in this dimension comprise a separate AI RMF audience who informs the primary audience. These AI actors may include trade associations, standards developing organizations, researchers, advocacy groups, environmental groups, civil society organizations, end users, and potentially impacted individuals and communities. These actors can:
-
assist in providing context and understanding potential and actual impacts;
-
be a source of formal or quasi-formal norms and guidance for AI risk management;
-
designate boundaries for AI operation (technical, societal, legal, and ethical); and
-
promote discussion of the tradeoffs needed to balance societal values and priorities related to civil liberties and rights, equity, the environment and the planet, and the economy.
Successful risk management depends upon a sense of collective responsibility among AI actors shown in Figure 3. The AI RMF functions, described in Section 5, require diverse perspectives, disciplines, professions, and experiences. Diverse teams contribute to more open sharing of ideas and assumptions about the purposes and functions of technology β making these implicit aspects more explicit. This broader collective perspective creates opportunities for surfacing problems and identifying existing and emergent risks.