1 Framing Risk
AI risk management offers a path to minimize potential negative impacts of AI systems, such as threats to civil liberties and rights, while also providing opportunities to maximize positive impacts. Addressing, documenting, and managing AI risks and potential negative impacts effectively can lead to more trustworthy AI systems.
In the context of the AI RMF, risk refers to the composite measure of an event’s probability of occurring and the magnitude or degree of the consequences of the corresponding event. The impacts, or consequences, of AI systems can be positive, negative, or both and can result in opportunities or threats (Adapted from: iso 31000:2018 ). When considering the negative impact of a potential event, risk is a function of 1) the negative impact, or magnitude of harm, that would arise if the circumstance or event occurs and 2) the likelihood of occurrence (Adapted from: omb Circular A-130:2016). Negative impact or harm can be experienced by individuals, groups, communities, organizations, society, the environment, and the planet.
While risk management processes generally address negative impacts, this Framework offers approaches to minimize anticipated negative impacts of AI systems and identify opportunities to maximize positive impacts. Effectively managing the risk of potential harms could lead to more trustworthy AI systems and unleash potential benefits to people (individuals, communities, and society), organizations, and systems/ecosystems. Risk management can enable AI developers and users to understand impacts and account for the inherent limitations and uncertainties in their models and systems, which in turn can improve overall system performance and trustworthiness and the likelihood that AI technologies will be used in ways that are beneficial.
The AI RMF is designed to address new risks as they emerge. This flexibility is particularly important where impacts are not easily foreseeable and applications are evolving. While some AI risks and benefits are well-known, it can be challenging to assess negative impacts and the degree of harms. Figure 1 provides examples of potential harms that can be related to AI systems.
AI risk management efforts should consider that humans may assume that AI systems work – and work well – in all settings. For example, whether correct or not, AI systems are often perceived as being more objective than humans or as offering greater capabilities than general software.
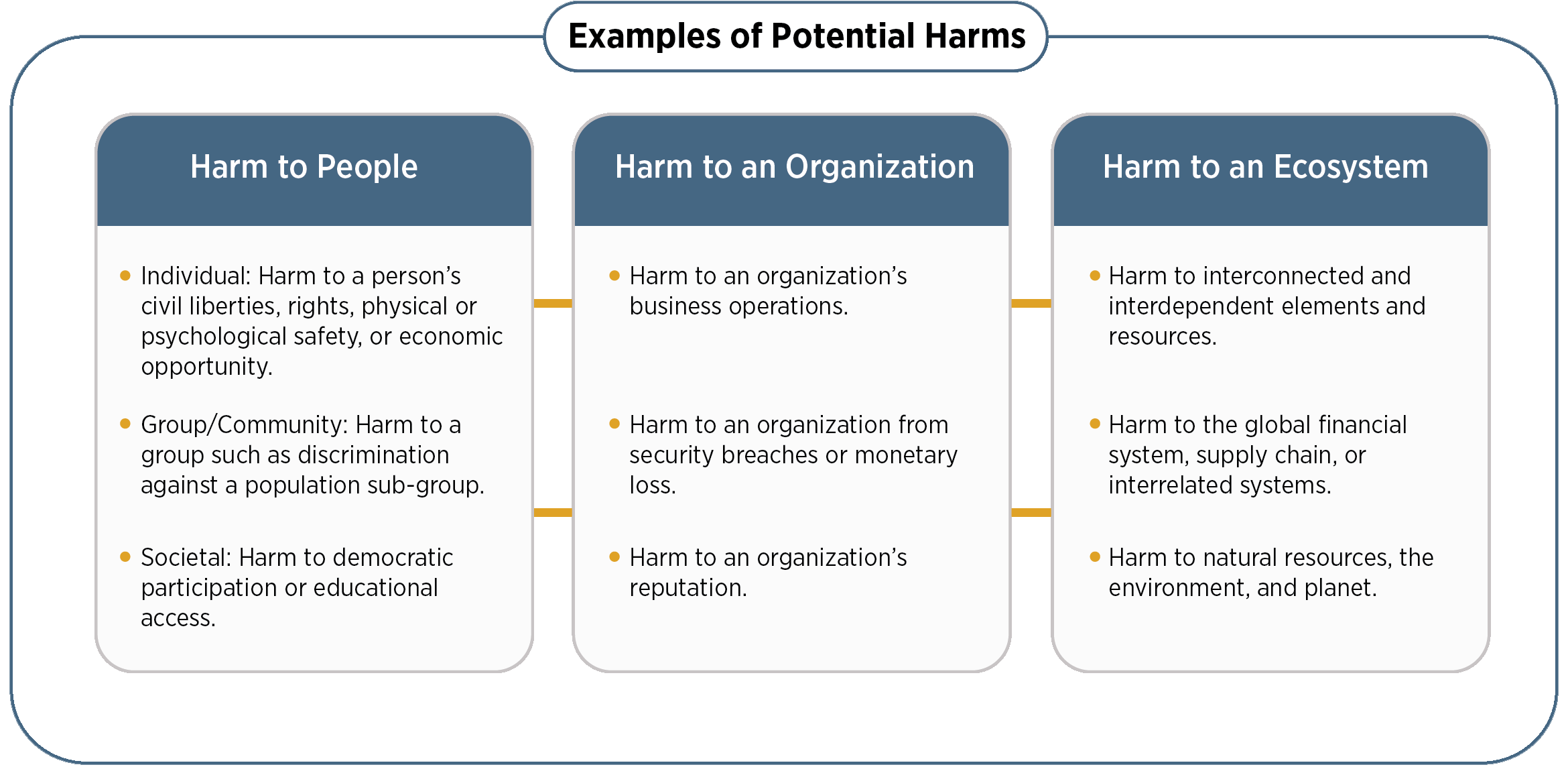
Several challenges are described below. They should be taken into account when managing risks in pursuit of AI trustworthiness.
1.1.1 Risk Measurement
AI risks or failures that are not well-defined or adequately understood are difficult to measure quantitatively or qualitatively. The inability to appropriately measure AI risks does not imply that an AI system necessarily poses either a high or low risk. Some risk measurement challenges include:
Risks related to third-party software, hardware, and data: Third-party data or systems can accelerate research and development and facilitate technology transition. They also may complicate risk measurement. Risk can emerge both from third-party data, software or hardware itself and how it is used. Risk metrics or methodologies used by the organization developing the AI system may not align with the risk metrics or methodologies uses by the organization deploying or operating the system. Also, the organization developing the AI system may not be transparent about the risk metrics or methodologies it used. Risk measurement and management can be complicated by how customers use or integrate third-party data or systems into AI products or services, particularly without sufficient internal governance structures and technical safeguards. Regardless, all parties and AI actors should manage risk in the AI systems they develop, deploy, or use as standalone or integrated components.
Tracking emergent risks: Organizations’ risk management efforts will be enhanced by identifying and tracking emergent risks and considering techniques for measuring them. AI system impact assessment approaches can help AI actors understand potential impacts or harms within specific contexts.
Availability of reliable metrics: The current lack of consensus on robust and verifiable measurement methods for risk and trustworthiness, and applicability to different AI use cases, is an AI risk measurement challenge. Potential pitfalls when seeking to measure negative risk or harms include the reality that development of metrics is often an institutional endeavor and may inadvertently reflect factors unrelated to the underlying impact. In addition, measurement approaches can be oversimplified, gamed, lack critical nuance, become relied upon in unexpected ways, or fail to account for differences in affected groups and contexts.
Approaches for measuring impacts on a population work best if they recognize that contexts matter, that harms may affect varied groups or sub-groups differently, and that communities or other sub-groups who may be harmed are not always direct users of a system.
Risk at different stages of the AI lifecycle: Measuring risk at an earlier stage in the AI lifecycle may yield different results than measuring risk at a later stage; some risks may be latent at a given point in time and may increase as AI systems adapt and evolve. Furthermore, different AI actors across the AI lifecycle can have different risk perspectives. For example, an AI developer who makes AI software available, such as pre-trained models, can have a different risk perspective than an AI actor who is responsible for deploying that pre-trained model in a specific use case. Such deployers may not recognize that their particular uses could entail risks which differ from those perceived by the initial developer. All involved AI actors share responsibilities for designing, developing, and deploying a trustworthy AI system that is fit for purpose.
Risk in real-world settings: While measuring AI risks in a laboratory or a controlled environment may yield important insights pre-deployment, these measurements may differ from risks that emerge in operational, real-world settings.
Inscrutability: Inscrutable AI systems can complicate risk measurement. Inscrutability can be a result of the opaque nature of AI systems (limited explainability or interpretability), lack of transparency or documentation in AI system development or deployment, or inherent uncertainties in AI systems.
Human baseline: Risk management of AI systems that are intended to augment or replace human activity, for example decision making, requires some form of baseline metrics for comparison. This is difficult to systematize since AI systems carry out different tasks – and perform tasks differently – than humans.
1.1.2 Risk Tolerance
While the AI RMF can be used to prioritize risk, it does not prescribe risk tolerance. Risk tolerance refers to the organization’s or AI actor’s (see Appendix A) readiness to bear the risk in order to achieve its objectives. Risk tolerance can be influenced by legal or regulatory requirements (Adapted from: iso guide 73). Risk tolerance and the level of risk that is acceptable to organizations or society are highly contextual and application and use-case specific. Risk tolerances can be influenced by policies and norms established by AI system owners, organizations, industries, communities, or policy makers. Risk tolerances are likely to change over time as AI systems, policies, and norms evolve. Different organizations may have varied risk tolerances due to their particular organizational priorities and resource considerations.
Emerging knowledge and methods to better inform harm/cost-benefit tradeoffs will continue to be developed and debated by businesses, governments, academia, and civil society. To the extent that challenges for specifying AI risk tolerances remain unresolved, there may be contexts where a risk management framework is not yet readily applicable for mitigating negative AI risks.
1.1.3 Risk Prioritization
Attempting to eliminate negative risk entirely can be counterproductive in practice because not all incidents and failures can be eliminated. Unrealistic expectations about risk may lead organizations to allocate resources in a manner that makes risk triage inefficient or impractical or wastes scarce resources. A risk management culture can help organizations recognize that not all AI risks are the same, and resources can be allocated purposefully. Actionable risk management efforts lay out clear guidelines for assessing trustworthiness of each AI system an organization develops or deploys. Policies and resources should be prioritized based on the assessed risk level and potential impact of an AI system. The extent to which an AI system may be customized or tailored to the specific context of use by the AI deployer can be a contributing factor.
When applying the AI RMF, risks which the organization determines to be highest for the AI systems within a given context of use call for the most urgent prioritization and most thorough risk management process. In cases where an AI system presents unacceptable negative risk levels – such as where significant negative impacts are imminent, severe harms are actually occurring, or catastrophic risks are present – development and deployment should cease in a safe manner until risks can be sufficiently managed. If an AI system’s development, deployment, and use cases are found to be low-risk in a specific context, that may suggest potentially lower prioritization.
Risk prioritization may differ between AI systems that are designed or deployed to directly interact with humans as compared to AI systems that are not. Higher initial prioritization may be called for in settings where the AI system is trained on large datasets comprised of sensitive or protected data such as personally identifiable information, or where the outputs of the AI systems have direct or indirect impact on humans. AI systems designed to interact only with computational systems and trained on non-sensitive datasets (for example, data collected from the physical environment) may call for lower initial prioritization. Nonetheless, regularly assessing and prioritizing risk based on context remains important because non-human-facing AI systems can have downstream safety or social implications.
Residual risk – defined as risk remaining after risk treatment (Source: iso guide 73) – directly impacts end users or affected individuals and communities. Documenting residual risks will call for the system provider to fully consider the risks of deploying the AI product and will inform end users about potential negative impacts of interacting with the system.
1.1.4 Organizational Integration and Management of Risk
AI risks should not be considered in isolation. Different AI actors have different responsibilities and awareness depending on their roles in the lifecycle. For example, organizations developing an AI system often will not have information about how the system may be used. AI risk management should be integrated and incorporated into broader enterprise risk management strategies and processes. Treating AI risks along with other critical risks, such as cybersecurity and privacy, will yield a more integrated outcome and organizational efficiencies.
The AI RMF may be utilized along with related guidance and frameworks for managing AI system risks or broader enterprise risks. Some risks related to AI systems are common across other types of software development and deployment. Examples of overlapping risks include: privacy concerns related to the use of underlying data to train AI systems; the energy and environmental implications associated with resource-heavy computing demands; security concerns related to the confidentiality, integrity, and availability of the system and its training and output data; and general security of the underlying software and hardware for AI systems.
Organizations need to establish and maintain the appropriate accountability mechanisms, roles and responsibilities, culture, and incentive structures for risk management to be effective. Use of the AI RMF alone will not lead to these changes or provide the appropriate incentives. Effective risk management is realized through organizational commitment at senior levels and may require cultural change within an organization or industry. In addition, small to medium-sized organizations managing AI risks or implementing the AI RMF may face different challenges than large organizations, depending on their capabilities and resources.